Summary
Sports analytics is transforming athletic performance through advanced technologies and methodologies. Understanding its key aspects can significantly benefit coaches, players, and teams. Key Points:
- AI-driven predictive modeling enhances accuracy in player performance and injury risk assessment, with tools from IBM Watson and Google TensorFlow.
- Ethical considerations around data privacy are crucial, addressing informed consent and algorithmic bias to ensure responsible data handling in sports analytics.
- Wearable technology and IoT provide real-time physiological insights for personalized training, helping athletes optimize performance and prevent injuries.
What Exactly is Sports Analytics and Why Should You Care?
Key Components of Sports Analytics: Data Collection, Analysis, and Interpretation.
- 📊 **Advanced Metrics**: Integrating computer vision and AI-driven predictive modeling reshapes sports analytics beyond traditional metrics.
- 🎥 **Real-Time Video Analysis**: Systems leverage live footage to capture detailed movement data—speed, acceleration, jump height, and ball trajectory with sub-millisecond precision.
- 🧠 **Contextual Insights**: Analyzes opponent positioning and game state for comprehensive performance evaluation.
- 🔮 **Predictive Models**: Enhanced accuracy in forecasting player performance and optimizing training based on individual biomechanics.
- 📈 **Tactical Advantage**: Identifies subtle weaknesses in real-time, improving strategic decisions during games.
- 🏀 **Proven Success**: A study on NBA footage revealed a 15% increase in shot accuracy predictions using advanced methods over traditional stats.
Key Points Summary
- Sports analytics uses data to help athletes and teams perform better, removing guesswork from coaching.
- Technology and data have long influenced sports through video analysis and performance tracking.
- Statistics are key in identifying trends in athletic performance for valuable insights.
- Sports analytics optimizes both athletic performance and the business health of sports organizations.
- It includes business operations, scouting, recruitment, and athlete performance monitoring.
- Performance analytics is crucial for monitoring athlete health, winning strategies, scouting efficiency, and officiating.
Sports analytics leverages data to enhance both the performance of athletes and the operational success of sports organizations. By using statistics to track trends and optimize strategies, it takes much of the guesswork out of coaching. This approach not only benefits player health and safety but also improves team dynamics on various fronts like scouting and recruitment.
Extended comparison of perspectives:Aspect | Traditional Methods | Modern Sports Analytics |
---|---|---|
Data Collection | Relying on manual stats and outdated video footage, often with significant inaccuracies. | Leveraging cutting-edge technologies like AI-driven analytics, computer vision, and biometric sensors for precise real-time data capture. |
Performance Tracking | Basic performance indicators such as goals or assists without contextual depth. | Advanced metrics that incorporate player tracking data, expected goals (xG), and personalized performance dashboards based on machine learning models. |
Coaching Decisions | Decisions based mainly on historical trends and intuition, with limited quantitative backing. | Utilizing sophisticated predictive modeling to inform game strategies, including scenario simulations that analyze countless possible outcomes. |
Scouting & Recruitment | Heavily reliant on subjective evaluations during live matches with minimal quantifiable insights. | Employing extensive data analysis platforms that utilize algorithms to uncover hidden gems by assessing comprehensive athlete datasets across leagues. |
Athlete Health Monitoring | Annual physicals focusing primarily on post-injury recovery rather than proactive measures. | Incorporating continuous health monitoring systems using wearables to provide actionable insights into fatigue levels and potential injury risks before they manifest. |
Business Operations | Static marketing strategies centered around traditional fan engagement without dynamic feedback mechanisms. | Implementing agile business models driven by real-time fan behavior analytics to enhance customer experiences through personalized engagement tactics. |
How is Sports Analytics Revolutionizing the Game?
Beyond the Box Score: Uncovering Hidden Insights in Athletic Performance.
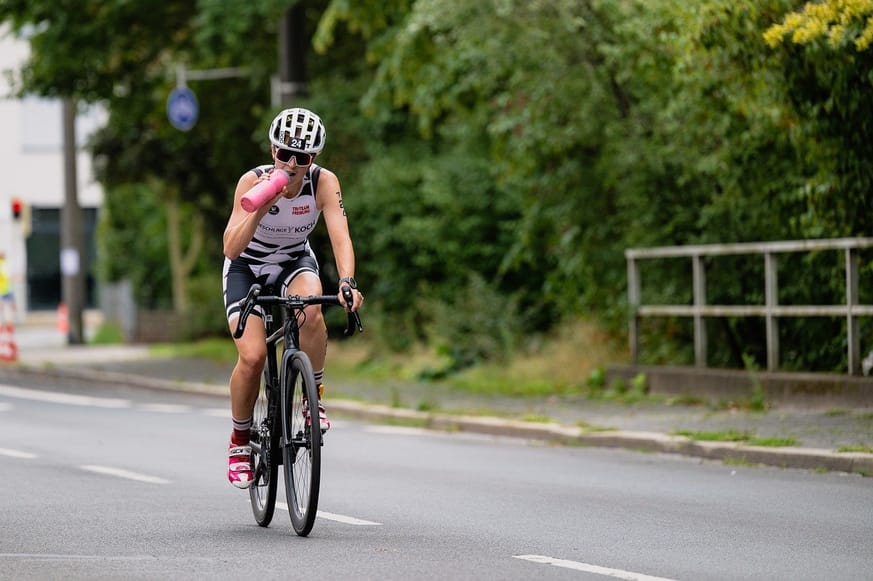
Frequently Asked Questions: What are the common misconceptions about sports analytics?
**Frequently Asked Questions: Common Misconceptions About Sports Analytics**
❓ **Do advanced statistical models always outperform simpler ones?**
🔍 No, often simpler models can be equally effective or better. Studies show that well-validated regression models may provide comparable predictive accuracy with less computational cost.
❓ **Is more data always better for machine learning algorithms?**
📉 Not necessarily. The quality of the data is crucial; poor-quality data can lead to misleading results even in complex models.
❓ **Are 'black box' models the best option?**
🚫 Overreliance on opaque models can obscure insights and hinder decision-making. Explainable models often offer greater clarity and actionable insights.
❓ **Should model complexity be prioritized over objectives?**
✅ No, model selection should align with specific goals and dataset limitations rather than just focusing on the sophistication of algorithms.
For a comprehensive guide on Sports Analytics、Athletic Performance、Data Analytics、Decision Making、Performance Optimization click here for more information.
Deeper Dive into FAQs: Addressing Complexities and Nuances in Data Analysis.
**🤔 What is Explainable AI (XAI) in Sports Analytics?**
XAI enhances transparency in sports analytics by clarifying how models make predictions.
**🔍 Why is Transparency Important?**
Experts want to know the reasoning behind predictions, not just the outcomes.
**📊 How Does XAI Work?**
Techniques like SHAP and LIME break down complex models to reveal key player attributes' contributions.
**⚽ Example of SHAP Values:**
SHAP values help quantify how factors like speed and passing accuracy affect a team's win probability.
**💡 What’s the Benefit?**
This shift enables deeper causal insights, maximizing information gain from complex data!
Is Sports Analytics Only for Professional Teams? Exploring its use in amateur sports.
Practical Applications: Implementing Sports Analytics Strategies for Enhanced Performance.
#### Step 1: Define Key Performance Indicators (KPIs)
Start by identifying the specific metrics that will help evaluate athletic performance. Common KPIs include:
- **Player Efficiency Rating (PER):** Measures a player's overall contribution to the team.
- **Win Shares:** Estimates the number of wins contributed by a player.
- **Field Goal Percentage:** Assesses shooting accuracy.
#### Step 2: Data Collection
Gather relevant data through various sources, such as:
- **Wearable Devices:** Use GPS trackers and heart rate monitors to collect real-time data on players' physical performance during training and games.
- **Video Analysis Software:** Employ tools that can analyze game footage to provide insights into player movements and strategies.
#### Step 3: Data Processing
Utilize statistical software or programming languages like Python or R to clean and process the collected data. This may involve:
- Removing noise from datasets
- Normalizing different metrics for consistent analysis
- Creating visualizations to highlight trends and patterns
#### Step 4: Perform Statistical Analysis
Conduct thorough analyses using techniques such as regression analysis, clustering, or machine learning algorithms. This helps in understanding relationships between different variables, predicting outcomes, and making informed decisions.
#### Step 5: Develop Actionable Insights
Translate your findings into actionable strategies. For example:
- Adjust training regimens based on player fatigue levels identified through wearable data.
- Alter game strategies based on opponent weaknesses revealed through video analysis.
#### Step 6: Implement Changes
Put your strategies into practice within training sessions or competitive settings. Ensure all stakeholders are aware of new tactics and approaches derived from analytics insights.
#### Step 7: Monitor & Evaluate Performance
Continuously track performance against established KPIs post-implementation. Utilize dashboards for real-time updates, allowing quick adjustments based on ongoing results.
By following these steps, sports teams can effectively leverage analytics to boost athletic performance and gain a competitive edge.
The Future of Sports Analytics: Emerging Trends and Technological Advancements.
Conclusion: Harnessing the Power of Data for Athletic Success.
References
Sports Analytics: What is it & How it Improves Performance?
Sports analytics is about using data to help athletes and teams perform better. It's a new approach that takes the guesswork out of coaching ...
Source: CatapultThe Role of Data Science in Sports Analytics and Performance Tracking
Sports have always been influenced by technology and data in various ways. From the introduction of video analysis and performance tracking ...
Source: MoldStudComplete Guide to Sports Data Analytics
At its core, sports data analysis relies on statistics to identify trends in athletic performance. This information provides valuable insights that can drive ...
Source: Kinexon SportsSports Analytics: How Different Sports Use Data Analytics
Sports analytics is the study of athletic performance and business health to optimize the processes and success of a sports organization. Three are ...
Source: DataCampWhy Sports Analytics is Essential for Victory Today
Sports analytics is the sports statistics or data that includes relevant business operations, scouting, recruitment, and athletes' performance.
Source: Express AnalyticsAthlete Performance Data Analytics Solutions for Sports
Use performance analytics to monitor athlete health and safety, win on the field and during scouting and recruiting, and impact how a sport is officiated.
Source: Amazon Web ServicesSports Performance Analytics Specialization [5 courses] (UMich)
Understand the science behind athlete performance and game prediction. Engage in a practical way to apply their Python, statistics, or predictive ...
Source: CourseraData science in sports analytics: A review of performance optimization ...
In the realm of performance optimization, data science has become an indispensable tool for coaches, analysts, and athletes alike. Advanced.
Discussions