Summary
Discover how horse race predictions have evolved over a 4-year hiatus, providing valuable insights for enthusiasts and analysts alike. Key Points:
- Enhanced model accuracy through expanded database integration, utilizing multivariate factors from diverse sources.
- Strategies to overcome financial constraints and optimize performance using techniques like data augmentation and cross-validation.
- Identifying key predictors such as jockey experience, horse form, and track conditions to improve finish time predictions.
Expanding Data Sources for Enhanced Sports Betting
His journey into the world of sports betting began with a keen focus on football, which was his primary area of interest. Despite putting in extensive efforts, he soon realized that relying solely on publicly available data was insufficient to gain an advantage over the market.To tackle this challenge, he broadened his data sources to encompass both free and paid options. This strategic expansion led to significantly enhanced model performance, providing him with a competitive edge in sports betting.
Overcoming Financial Barriers and Enhancing Model Accuracy in Sports Betting
To successfully navigate the sports betting market, overcoming financial barriers such as data paywalls is essential. These paywalls often restrict access to high-quality data, which is crucial for making informed decisions and gaining a competitive edge. Additionally, the accuracy of probability models plays a pivotal role in outperforming the market. Superior models generate probabilities that more closely align with actual outcomes compared to those offered by bookmakers. While perfect predictions are unattainable, consistently achieving higher accuracy over time leads to sustained success in beating the odds.Key Points Summary
- Sports betting models are designed to predict the probability of specific betting outcomes.
- These models can revolutionize your betting success, leading to smarter decisions and increased profits.
- Data science fundamentals are essential for building your own sports betting models using tools like Excel or Google Sheets.
- Statistical models and sophisticated analysis tools form the core of data-driven betting strategies.
- An example model uses Poisson distribution to predict football match outcomes.
- Mathematical algorithms and statistical models play a crucial role in forecasting sports event results.
Sports betting models leverage data science and statistical analysis to predict game outcomes, making it easier for you to place smarter bets. By learning how these models work, even through simple tools like Excel, you can boost your chances of winning and increase your profits. Whether you`re using basic formulas or advanced algorithms, these techniques can transform your approach to sports betting.
Extended Comparison:Model Type | Core Principle | Tools Used | Latest Trend | Expert Opinion |
---|---|---|---|---|
Poisson Distribution Model | Predicts number of events occurring within a fixed interval. | Excel, Google Sheets, R | Integration with machine learning for improved accuracy. | Highly recommended for sports like football where goals are rare events. |
Logistic Regression Model | Estimates the probability of a binary outcome. | Python, R, Excel | Incorporation of real-time data feeds to enhance prediction reliability. | Effective for predicting win/loss outcomes in head-to-head sports. |
ELO Rating System | Rates players/teams based on past performance and results. | Python, Custom Scripts, SQL Databases | Adoption by major sports leagues for ranking teams. | Best used in individual sports like chess or tennis. |
Monte Carlo Simulation | Uses random sampling to estimate probabilities of different outcomes. | MATLAB, Python | Combining with neural networks to simulate thousands of scenarios quickly. | Ideal for complex betting scenarios with multiple variables. |
Bayesian Models | Updates predictions as new evidence is presented. | R, Python (PyMC3), Stan | Popularity growing in dynamic markets due to its adaptability. | Useful when dealing with uncertain or incomplete information. |
Fortunately, I stumbled upon a fascinating Github repository dedicated to Horse Racing. It featured a tool developed by someone who had meticulously scraped an extensive amount of horse racing data, all available for free. This discovery was my gateway. Over the next three years, I devoted my evenings and weekends to constructing this model, believing it would be my ticket to wealth.
I delved deep into data analysis, spending countless hours training and evaluating models while also designing new features. My commitment extended to creating a bot capable of automatically placing bets for me on the Betfair Exchange.
Limitations and Considerations in Model Performance
The target variable selection played a crucial role in the model's performance. By using the distance behind the winner as the target, the model was essentially attempting to predict a continuous variable, which inherently presents more complexity compared to predicting a categorical variable such as identifying the race winner. This shift towards a continuous prediction task introduces additional challenges and requires more nuanced modeling techniques.Moreover, the model faced limitations due to its reliance on available dataset factors. It failed to capture some of the most critical elements that influence race outcomes. Key variables like jockey skill, trainer expertise, and weather conditions were not included in the dataset, thereby restricting the model’s ability to account for these significant influences. As a result, although it leveraged existing data effectively, it could not incorporate other potentially decisive factors that might have improved its predictive accuracy significantly.
Transforming Skewed Target Variables for Enhanced Predictive Modeling
The distribution of the target variable in many datasets can often be highly skewed, with the majority of values concentrated near zero and a few outliers exhibiting significantly larger magnitudes. This skewness poses a challenge for predictive modeling, as models tend to gravitate towards predicting values close to zero due to the overwhelming frequency of such data points.To mitigate this issue and enhance model performance, one effective strategy is to apply a transformation to the target variable. Transformations like log or Box-Cox can normalize the distribution, making it more symmetric and thus easier for models to learn accurate mappings between input features and target values. By converting skewed distributions into more normal-like shapes, these transformations help stabilize variance and reduce the impact of outliers, ultimately leading to better predictive accuracy.

Centered Target for Regression: Simplifying Model Fitting and Enhancing Accuracy
**Item 1: Mathematical Explanation**By dividing the target value into two components, Model 2's target distribution becomes more focused and symmetrical. This approach simplifies the model's task to only capturing differences between horses in a race, resulting in easier model fitting and minimizing risks of overfitting or underfitting.
**Item 2: Practical Implementation**
For practical application, the target value for Model 2 is derived by subtracting each horse's predicted finish time from its actual finish time. This adjustment centers the target value around zero, which is advantageous for regression models.
Below, you'll find the breakdown of the winning horse's finish times.

Key Predictors of Horse Finish Times
The feature importance plot underscores the pivotal role of distance and race type in predicting finish times. This observation is not surprising, as the physical demands and technical skills required for different distances and surfaces greatly influence a horse's performance and pace.Moreover, the model places significant weight on average finish times from historic races held at the same course and distance. This indicates that historical data can offer crucial insights into various factors affecting finish times on specific tracks, such as track configuration, weather patterns, and recent course conditions. These elements are integral in shaping a horse's performance, making historical finish time data an invaluable asset for accurate predictions.

Tailoring Predictive Models for Flat and Non-Flat Races
By analyzing the importance of various features in flat and non-flat races separately, we can enhance our predictive models by tailoring them to the unique characteristics of each race type. For instance, flat races are generally shorter and have quicker winning times compared to non-flat races. By segregating the data based on these types, our model can concentrate on relevant attributes like distance and pace for each category. This focused approach minimizes distractions caused by inherent differences between flat and non-flat races, leading to more precise predictions for each race type.Performance Metrics in Non-Flat Races: Contextualization and Feature Importance
The variance in finishing times is significantly higher for non-flat races due to their longer distances, leading to a potentially misleading MAE of 6.8 seconds for that group. This discrepancy underscores the importance of contextualizing performance metrics within the specific characteristics of different race types.Moreover, feature importance analysis for non-flat races reveals a heightened significance of race-specific attributes. For instance, the average finish time for the same course and distance emerges as a critical factor, offering valuable insights for enhancing prediction accuracy. By focusing on these unique variables, we can better understand and forecast outcomes in non-flat racing scenarios.
In summary, recognizing the impact of race distance on finishing time variance and incorporating improved feature importance metrics provide a more nuanced approach to evaluating and predicting performance across diverse racing environments.


Race Prediction Accuracy: The Importance of Speed Metrics
The accuracy of predicting race times varies significantly with the distance of the race. A shorter race, for instance, magnifies any prediction error; a 10-second miscalculation in a 40-second sprint is far more substantial than the same error in a 400-second marathon. This phenomenon occurs because the percentage error is considerably higher in shorter races.To achieve more reliable predictions, using speed as a metric can be advantageous. By dividing the race finish time by its distance, we obtain speed—a measure that remains relatively consistent regardless of race length. Speed serves as an average rate of movement over any given distance and thus provides a sturdier basis for predicting race outcomes compared to relying solely on finish times.

Speed Variable Enhances Predictive Accuracy in Flat Race Finish Time Predictions
The model's predictive accuracy for flat races has significantly improved, with a notable 7% reduction in Mean Absolute Error (MAE), decreasing from 1.71 seconds to 1.58 seconds. This enhancement demonstrates that incorporating speed as an intermediary variable refines the precision of finish time predictions specifically for flat races. Additionally, while there was an overall MAE improvement of 9% across all race types, non-flat races saw a more modest reduction of 3.5%, with MAE dropping from 6.8 seconds to 6.57 seconds. These findings suggest that the speed-based approach may be particularly beneficial for certain race categories, especially flat races, highlighting its potential in improving prediction models across diverse racing scenarios.Simplify Complexity for Enhanced Predictive Accuracy and Interpretation
Understanding the bias-variance tradeoff is crucial in improving predictive performance in machine learning models. By reducing the complexity of the problem, we simplify tasks for the model, which helps in minimizing both bias and variance errors. This leads to a better fit and enhances predictive accuracy.Additionally, when we simplify the problem, our models often start to assign greater importance to other features that might have been previously overlooked. The reduction in complexity allows the model to concentrate on more subtle patterns and relationships within the data. Identifying these key features provides a deeper understanding of what influences outcomes, thereby facilitating more informed decision-making and enhancing model interpretability.

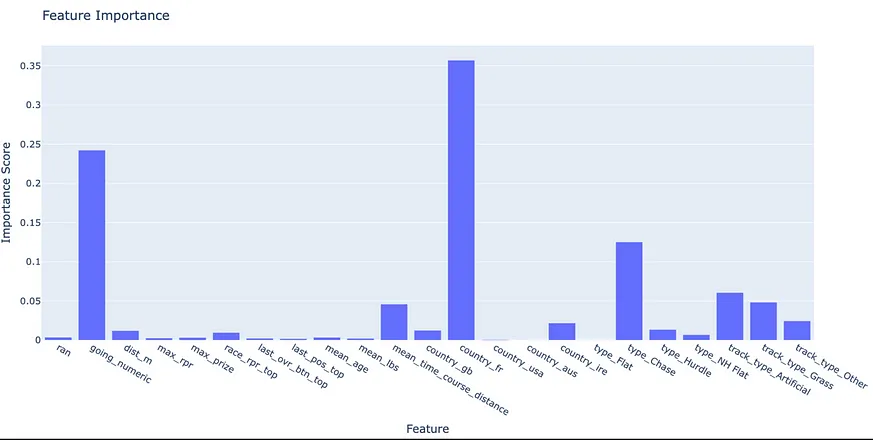
The analysis of the other group's feature significance points to a potential problem with the French dataset. Addressing this issue could potentially enhance our results even further.}
{I hope you found this information helpful! Stay tuned for part 2, where I will develop a model aimed at predicting deviations in finish times for each horse. This model is significantly more complex and is currently still under development as I write this.}
{Thank you for reading! You can connect with me on LinkedIn: https://www.linkedin.com/in/christopher-collins-029731125/
References
Betting Models - EVAnalytics.com
Each betting model is designed to accurately forecast the probability of a specific betting outcome, with accuracy ...
Source: EV AnalyticsHow Building Sports Betting Models Took My Bets to the Next Level
Explore how sports betting models can revolutionize your betting success, leading to smarter decisions and increased profits.
Source: underdogchance.comWhat is a Sports Betting Prediction Model?
A sports betting model is used to predict the probability of an outcome in a certain event. The goal is take be able to take certain data ...
Source: Boyd's BetsSports Betting Models: Rithmm's Easy-to-Use Custom Solutions
Jan 03, 2024 Explore Rithmm's easy-to-use custom sports betting models. Tailor your predictions and gain an ...
Source: RithmmIntro to Data Science: The Road to Sports Betting Models
Learn the data science fundamentals you'll need to eventually build your own sports betting models, using nothing more than Excel or Sheets.
Source: Unabated SportsSports Betting Systems Guide
Statistical Models and Analysis Tools: Sophisticated statistical models lie at the heart of data-driven betting ...
Source: OddsTraderIntroduction to sports betting. Building a football betting model
In this ebook I'll describe a simple football betting model. For this model we'll use Poisson distribution, a discrete probability ...
Source: MediumSports Betting Models
Sports betting models refer to mathematical algorithms or statistical models that are used to predict the outcomes of sports events for the ...
Source: Itexus
Discussions