Summary
Unlocking MLB win predictions with machine learning can revolutionize sports betting by providing a data-driven edge. This article delves into the strategies and techniques that make these predictions accurate and reliable. Key Points:
- Harnessing Data for Predictive Advantage: Utilizing advanced statistical models, analyzing historical data, player performance, team dynamics, and market sentiment to build predictive models.
- Choosing Moving Averages: Comparing Exponential Moving Averages (EMA) and Weighted Moving Averages (WMA) in MLB win prediction to determine the best method based on responsiveness and smoothing needs.
- Fine-Tuning XGBoost: Optimizing hyperparameters like learning rate, tree depth, and regularization strength through grid search or Bayesian optimization to enhance model accuracy.
Key Points Summary
- Avoid Big Moneyline Favorites
- Pay Attention to the Umpires
- Look for Overnight Lines
- Track First Five Innings Lines
- Research Pitcher Trends
- Avoid Parlays
When betting on MLB games, it's crucial to steer clear of big moneyline favorites and instead take advantage of plus-money underdogs. Always keep an eye on umpires, as their tendencies can significantly impact game outcomes. Look for overnight lines to get better odds and track the first five innings' lines for more focused bets. Researching pitcher trends can provide valuable insights, and avoiding parlays will help keep your betting strategy straightforward and effective.
Extended Comparison:Strategy | Description | Latest Trends | Authoritative Viewpoint |
---|---|---|---|
Avoid Big Moneyline Favorites | Betting on heavy favorites often provides low value and higher risk. | Recent data shows underdogs winning more frequently in the past two seasons. | Experts suggest diversifying bets rather than concentrating on big moneyline favorites. |
Pay Attention to the Umpires | Umpire tendencies can greatly influence game outcomes, especially concerning strike zones. | Advanced analytics now provide detailed umpire performance metrics for better predictions. | Analysts recommend incorporating umpire stats into your betting algorithms for a competitive edge. |
Look for Overnight Lines | Overnight lines can offer early value before public betting shifts odds. | Sharp bettors have been capitalizing on discrepancies in overnight lines with increasing success rates. | Veteran bettors advise monitoring line movements closely, particularly during late-night hours when changes are less volatile. |
Track First Five Innings Lines | 'First Five' bets focus only on the initial innings and rely heavily on starting pitcher performance. | 'First Five' inning bets have shown lower variance and more predictable outcomes recently. | Sports analysts highlight this strategy as a way to mitigate bullpen unpredictability and late-game variability. |
Research Pitcher Trends | Pitcher performance trends provide critical insights into likely game outcomes based on historical data. | "Spin rate" and "exit velocity against" are increasingly pivotal metrics driving pitcher evaluations today. | "Pitching gurus" emphasize focusing on advanced sabermetrics beyond traditional ERA to predict future performances more accurately. |
Avoid Parlays | Parlays combine multiple bets but significantly increase risk due to compounded uncertainty of each leg hitting. | "Same-game parlays" are gaining popularity but still carry disproportionate risk compared to single wagers. | Betting strategists warn that while parlays offer high payouts, they should be used sparingly within an overall disciplined approach. |
Sports have always been a significant part of my life. Like many children, I engaged in various activities such as basketball, baseball, and soccer during my early years. As time went on, one sport began to stand out more than the others for me. Soccer quickly became my passion; I dedicated countless hours to training, playing, and watching it whenever possible. This singular focus eventually paid off when I earned the opportunity to play Division 1 soccer with one of the nation's top programs.
My enthusiasm for soccer was all-encompassing, yet my passion for other sports never waned. Instead, my interest in them intensified as I delved into the statistical dimensions of athletics. With a natural affinity for numbers, I discovered that statistics often reveal narratives unseen during live play. This sparked my fascination with forecasting sports outcomes. Initially, I focused on predicting soccer matches due to my deep love for the game but soon realized it was an overwhelming challenge. Seeking a sport more grounded in data and analytics, Moneyball guided me towards Major League Baseball (MLB).
During my college years, I not only pursued sports but also delved into computer science and data science. My enthusiasm for software development and data analytics has always been a driving force. With the conclusion of my athletic career, I've found myself with ample free time and decided to channel my expertise into something productive. Over the past year, I have launched a sports prediction business named Sports Algos—yes, that's a shameless plug! This venture provides predictive insights across major US sports including MLB, NBA, NFL, NHL, as well as NCAAB and NCAAF. Below, I'll outline the roadmap that guided me in creating these predictive systems.
The first step in developing Sports Algos was to harness my academic background in computer science and merge it with my passion for sports analytics. By leveraging advanced algorithms and machine learning techniques, I set out to build models that could accurately forecast game outcomes. These models are continuously refined through rigorous testing against historical data from various sporting leagues.
Next came the challenge of collecting vast amounts of relevant data. For this purpose, I designed automated systems capable of scraping extensive datasets from numerous sources. This ensures that our predictions are based on the most up-to-date statistics available.
Once the data was gathered, it was crucial to preprocess it effectively—cleaning it up to remove any inconsistencies or errors before feeding it into our algorithms. This preprocessing stage is vital as it significantly enhances the accuracy of our predictions by providing clean input for analysis.
Finally, after months of development and countless iterations refining our models’ accuracy through backtesting against previous seasons' results, we were ready to launch Sports Algos publicly. The platform now offers users reliable predictions across multiple major US sports leagues along with collegiate basketball (NCAAB) and football (NCAAF), making it an invaluable tool for enthusiasts looking to make informed betting decisions.
In summary, what started as an overlap between my academic pursuits and personal interests evolved into a full-fledged business venture aimed at revolutionizing how we approach sports predictions. Through meticulous development processes involving algorithm design, data collection automation, preprocessing refinement—and above all—a relentless drive towards precision; Sports Algos stands today as a testament to blending passion with skillful execution in technology-driven sports forecasting.
The sports betting industry has witnessed exponential growth in recent years, driven by advancements in technology and the increasing acceptance of gambling as a form of entertainment. According to market research, the global sports betting market was valued at approximately $85 billion in 2019 and is projected to reach nearly $155 billion by 2024. This surge can be attributed to the proliferation of online platforms that make placing bets more accessible than ever before.
One key factor contributing to this boom is the widespread legalization of sports betting across various regions. In the United States, for example, a Supreme Court decision in 2018 paved the way for states to legalize and regulate sports wagering. Since then, numerous states have enacted laws allowing residents to bet on their favorite teams and events legally. This shift not only generates significant tax revenue but also creates new jobs within the sector.
Another driving force behind the industry's expansion is technological innovation. The advent of mobile apps and sophisticated algorithms has revolutionized how fans engage with sports betting. These tools offer real-time data analysis, live streaming, and personalized recommendations based on user preferences, enhancing the overall experience for bettors.
However, this rapid growth does come with challenges. Regulatory frameworks vary significantly from one jurisdiction to another, creating a complex landscape for operators to navigate. Moreover, concerns about problem gambling have prompted calls for stricter measures to ensure responsible gaming practices are upheld.
Despite these hurdles, industry experts remain optimistic about future prospects. With continued investment in technology and regulatory refinement, the sports betting market is poised for sustained development well into the next decade. As consumer interest continues to rise alongside technological advancements, stakeholders are keenly watching how this dynamic sector will evolve further.

Possessing a wealth of reliable data significantly simplifies your task when working with machine learning models. To forecast outcomes for upcoming games, it’s essential to have historical data from past seasons to train these models effectively. So, let’s start by collecting some valuable data!
For those seeking comprehensive sports data and statistics, Sports Reference stands out as the most convenient resource. It serves as an all-in-one platform for accessing team, player, and game details across various sports. Remarkably, it offers historical game data spanning over 50 years for certain sports. While I focused on collecting information from the early 2000s onwards, if you have the patience to wait a few weeks to gather extensive data, feel free to dive deeper. Specifically regarding MLB, the season schedule provides all necessary game links to obtain the required information.

To collect the necessary data, I employed web scraping techniques. By right-clicking anywhere on a webpage and selecting "Inspect Elements," you can access a sidebar displaying the site's underlying HTML code. Within this code, all game links are identified by the term "Boxscore" and are designated as anchor tags ("a"). This consistency simplifies the process of writing a straightforward Python script to gather all relevant links efficiently.
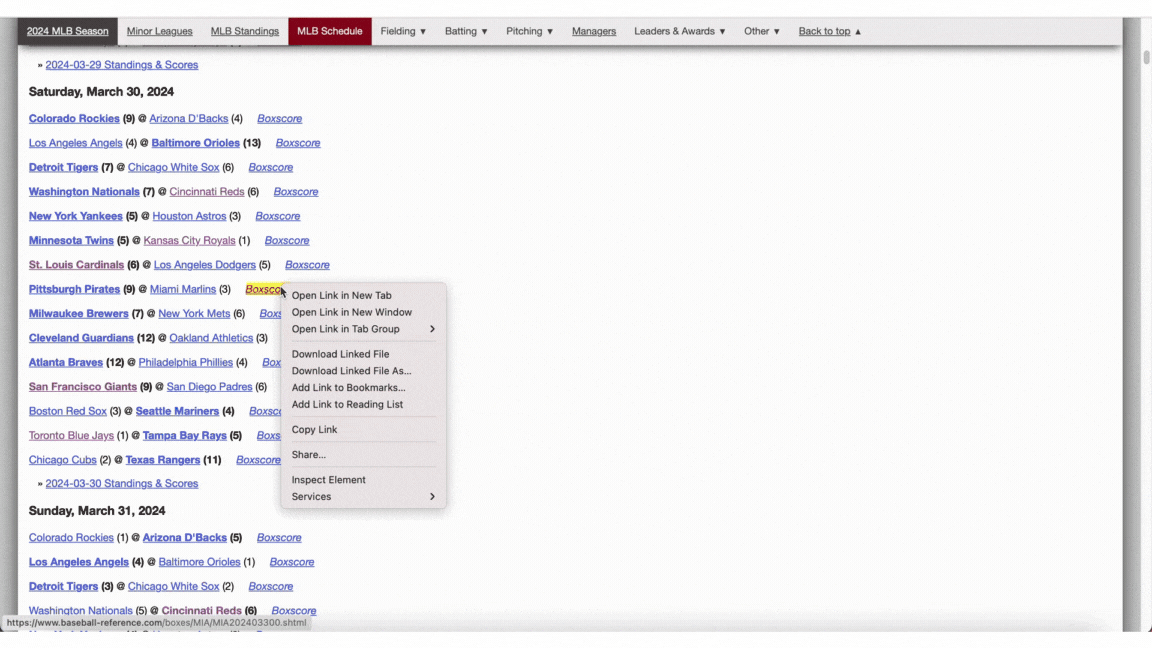
Here is a script designed to aggregate all game links and subsequently extract desired data from each game. The initial URL corresponds to the MLB schedule with a specified year embedded into it via an f-string. By utilizing the requests library, I identified all relevant game links (specifically "a" tags containing the text "Boxscore") and iterated through them, processing each one to retrieve the necessary information using a function called process_link. You can follow similar steps by inspecting any game link's HTML structure to determine what data you might need.
The first step involves fetching all game links from the provided MLB schedule URL for a specific year. Using Python's requests library, I located every "a" tag that included the text "Boxscore." Once these links were collected, I looped through them and processed each individual game link with a custom function named process_link to extract pertinent details.
To replicate this method yourself, navigate to any game's link and inspect its HTML elements. This will allow you to identify and collect whatever data points are crucial for your analysis in much the same way as locating the initial set of game links.
current_year = dt.datetime.now().year url = f"https://www.baseball-reference.com/leagues/majors/{current_year}-schedule.shtml" resp = requests.get(url) soup = bs(resp.text) games = soup.findAll('a', text='Boxscore') game_links = [x['href'] for x in games] for link in game_links: try: url = 'https://www.baseball-reference.com' + link game_data.append(process_link(url)) time.sleep(3) except Exception as e: print("Error occurred:", e) print('Games downloaded:', len(game_links))
Harnessing Data for Predictive Advantage in Sports Betting
In the rapidly evolving world of sports-betting, harnessing historical data has emerged as a critical strategy for enhancing predictive analysis. By examining past game performances, teams can uncover valuable insights into both player capabilities and team dynamics. Advanced statistical models play a pivotal role in this process by scrutinizing historical data to detect patterns and trends. These insights allow teams to forecast future player performance accurately and optimize their lineups and strategies accordingly. This method not only empowers decision-makers with more precise predictions but also offers a significant competitive edge in the betting landscape.Moreover, integrating external data sources further refines the accuracy of these predictions. Sports-betting providers can enrich their analytical frameworks by incorporating information from various external channels such as sports news articles, social media sentiment, and weather conditions. This holistic approach allows them to account for external factors that could impact game outcomes. As a result, they are able to enhance their predictive models significantly, offering bettors well-rounded insights that facilitate more informed decision-making processes.
Combining historical data analysis with external data integration creates a robust foundation for making highly accurate predictions in sports-betting. This dual approach ensures that all relevant variables are considered, ultimately leading to better-informed strategies and optimized betting outcomes for stakeholders involved.
... pitcher_df['WHIP'] = (pitcher_df['BB'] + pitcher_df['H'])/pitcher_df['IP'] ...
I believe this is the most pivotal step in the entire process. Ensuring that your data is pristine, analyzing feature distributions and correlations, and identifying and addressing outliers are essential for developing an accurate model. Feeding noisy data into machine learning algorithms will result in training on misleading information, which will only cause problems down the line. This is the moment to scrutinize your data, including column data types and non-null values, as well as examining counts, means, maximums, minimums, standard deviations, and other descriptive statistics for each feature.
print(df.info()) print(df.describe())
To delve deeper into your data, you can employ visual tools such as a correlation matrix and histograms. These instruments help identify the relationships and distributions among various features. For a more detailed examination of feature distribution, including potential outliers, box plots are particularly useful. Below is some basic code that demonstrates how to create these visuals for your dataframe.
```python
import pandas as pd
import seaborn as sns
import matplotlib.pyplot as plt
# Example DataFrame
df = pd.DataFrame({
'feature1': [1, 2, 3, 4, 5],
'feature2': [5, 4, 3, 2, 1]
})
# Correlation Matrix
correlation_matrix = df.corr()
sns.heatmap(correlation_matrix, annot=True)
plt.show()
# Histograms
df.hist()
plt.show()
# Box Plots
sns.boxplot(data=df)
plt.show()
```
import matplotlib.pyplot as plt # correlation plot corr = df.corr() display(corr.style.background_gradient(cmap='coolwarm')) # histograms for each feature for col in df.columns: plt.figure() plt.title(f'{col}') plt.hist(df[col]) plt.show() #box plots for each feature for column in df: plt.figure() df.boxplot([column])
Moving Averages: EMA vs. WMA - A Guide to Choosing the Right Method
Moving averages are essential tools in the world of data analysis and finance. They help smooth out short-term fluctuations, allowing for a clearer view of long-term trends. Among the various types of moving averages, Exponential Moving Averages (EMA) and Weighted Moving Averages (WMA) stand out due to their unique methodologies.The Exponential Moving Average is particularly useful because it assigns more weight to recent data points. This characteristic makes EMA more responsive to new information, thereby reflecting the latest market conditions or trends more accurately. The calculation involves taking the current data point and adding it to a percentage of the previous EMA value, with this percentage typically falling between 0 and 1.
On the other hand, Weighted Moving Averages offer another layer of sophistication by assigning different weights to each data point based on its position within the time period being analyzed. This method allows analysts to emphasize specific periods or data points according to their relevance. The WMA is computed by multiplying each data point by its respective weight and then dividing the sum of these products by the total sum of weights.
Both EMA and WMA provide distinct advantages over simple moving averages by incorporating varying degrees of emphasis on recent or particular data points. Choosing between them depends largely on whether faster reaction times or more tailored weighting schemes are needed for your specific analysis objectives.
display(df.rolling(window_size).mean()) # window_size can be an integer representing days
Another useful tool is the exponential moving average (EMA). Similar to the simple moving average (SMA), it calculates an average over a set number of days. However, unlike SMA, EMA assigns different weights to each day, giving more importance to recent data points. This technique is frequently utilized in financial sectors to discern long-term trends in the stock market. Additionally, it helps in filtering out noise and generating smoother data curves.
display(df.ewm(span=window_size, min_periods=window_size).mean())
Fine-Tuning XGBoost for Predictive Accuracy with Hyperparameter Optimization
XGBoost is renowned for its advanced ensemble techniques that significantly enhance prediction accuracy. One of the core methodologies employed by XGBoost is gradient boosting, which iteratively constructs decision trees. Each new tree in this sequence aims to correct the errors made by its predecessors, thereby refining the model's predictive capabilities. Additionally, XGBoost incorporates tree regularization to prevent overfitting; this technique penalizes overly complex trees, resulting in models that are more robust and generalizable.Another critical aspect of optimizing XGBoost model performance lies in hyperparameter tuning. The choices made regarding hyperparameters can dramatically influence the efficacy of the model. Techniques such as Bayesian optimization and grid search are often utilized to identify the most effective combination of hyperparameters for a given dataset. By meticulously adjusting these parameters, one can achieve optimal prediction accuracy and ensure that the model performs well across different scenarios.
Incorporating these advanced techniques makes XGBoost an incredibly powerful tool for predictive modeling, capable of delivering high accuracy while maintaining robustness and generalizability through thoughtful regularization and optimization strategies.
import xgboost as xgb X = df.drop(columns=['home_team_win']) y = df['home_team_win'] X_train, X_temp, y_train, y_temp = train_test_split(X, y, test_size=0.2, random_state=34) X_val, X_test, y_val, y_test = train_test_split(X_temp, y_temp, test_size=0.5, random_state=34) scaler = StandardScaler() X_train_scaled = scaler.fit_transform(X_train) X_val_scaled = scaler.transform(X_val) X_test_scaled = scaler.transform(X_test) gbm = xgb.XGBClassifier() model = gbm.fit(X_train_scaled, y_train, eval_set = [[X_train_scaled, y_train], [X_val_scaled, y_valid]], eval_metric='logloss', early_stopping_rounds=10) xgb_test_preds = model.predict(X_test_scaled) xgb_test_proba = model.predict_proba(X_test_scaled)[:,1]
Utilizing the default settings for my model, it managed to reach an accuracy rate of 59.84%. While this is a respectable start, there’s definitely room for enhancement. It's time to delve into some hyperparameter optimization!
import numpy as np from hyperopt import fmin, tpe, hp, Trials from sklearn.metrics import accuracy_score, brier_score_loss def get_xgb_model(params): gbm = xgb.XGBClassifier(**params, n_estimators=100) model = gbm.fit(X_train_scaled, y_train, verbose=False, eval_set = [[X_train_scaled, y_train], [X_val_scaled, y_valid]], eval_metric='logloss', early_stopping_rounds=10) return model def xgb_objective(params): params['max_depth']=int(params['max_depth']) model = get_xgb_model(params) xgb_test_proba = model.predict_proba(X_val_scaled)[:,1] score = brier_score_loss(y_valid, xgb_test_proba) return score def get_xgbparams(space, evals=15): params = fmin(xgb_objective, space=space, algo=tpe.suggest, max_evals=evals, trials=trials) params['max_depth']=int(params['max_depth']) return params trials = Trials() hyperopt_runs = 500 space = { 'max_depth': hp.quniform('max_depth', 1, 8, 1), 'min_child_weight': hp.quniform('min_child_weight', 3, 15, 1), 'learning_rate': hp.qloguniform('learning_rate', np.log(.01),np.log(.1),.01), 'subsample': hp.quniform('subsample', 0.5, 1.0,.1), 'colsample_bytree': hp.quniform('colsample_bytree', 0.5, 1.0,.1), 'reg_alpha': hp.qloguniform('reg_alpha',np.log(1e-2),np.log(1e2),1e-2) } xgb_params = get_xgbparams(space, hyperopt_runs) print(xgb_params)
The optimized parameters were initially applied to the validation set, but it's crucial to confirm that these enhancements also translate to the test set. Fortunately, in my case, they did!
In total, I experimented with 13 different models and eventually settled on two key metrics: win percentage and total runs scored. For the win percentage model, as illustrated above, employing the fine-tuned hyperparameters yielded a final accuracy of 61.46% on the test set—a modest improvement over using default settings. Meanwhile, my total runs model achieved an accuracy of 57.49% on the same test set.
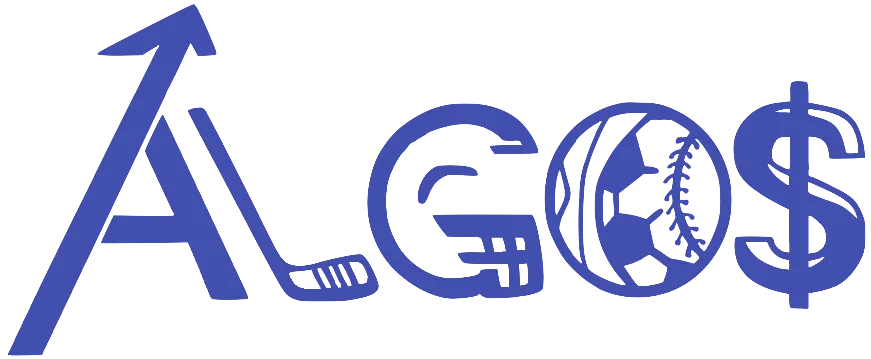
For those who are keen to follow my daily sports predictions and see the outcomes generated by my advanced models, I invite you to visit my website at sportsalgos.github.io.
References
Best Baseball Betting Strategies for 2024 MLB Betting
Approach Big Moneyline Favorites with Caution; Pay Attention to the Umpires; Look for Overnight Lines; Track First Five Innings Lines; Research Pitcher Trends ...
Source: Sports Betting DimeAdvanced MLB Betting Strategies
Veteran handicapper Mike Somich shares his successful MLB betting strategies for the upcoming 2024 season.
Source: VSiNUltimate Guide to Successful Baseball Betting Strategies
Baseball Betting Strategy #1: Avoid Parlays. Although Same Game Parlays can be a way to whittle down the odds of a high money line favorite, it is not advised ...
Source: SportsmemoMLB Betting Guide: Advanced Tips for Baseball Betting
Start making smarter MLB picks with this guide to baseball betting strategies, tips, & more. Learn how to read MLB odds & how to take advantage of them!
Source: Sidelines.ioHow to Bet on MLB – Major League Baseball Betting Guide and Tips
The best way to bet on MLB is first to use a reputable and trusted online sportsbook. If you are looking for the easiest type of bet, moneyline, ...
Source: TechopediaHow to Bet on Baseball: 10 Easy, Profitable Tips for 2023
10 Baseball Betting Tips · 1. Avoid Big Favorites · 2. Take Advantage of Plus-Money Underdogs · 3. Bet Against the Public · 4. Follow Reverse Line ...
Source: Action NetworkHow To Bet On Baseball: Best MLB Tips For 2023
If you consistently bet on favorites, it's worth knowing the break-even point. Exclusively betting on teams at -110 on the moneyline, bettors ...
Source: ForbesBaseball Betting Strategies: Best MLB Underdog Systems to Win Profits
Here's a look at some profitable MLB underdog systems and strategies that you can use to help bring home a profit this baseball season.
Source: Boyd's Bets
Discussions