Summary
The article explores how data science is transforming NFL talent evaluation through advanced techniques that enhance player safety and team performance. Key Points:
- Data science quantifies the financial impact of player injuries, helping NFL teams assess costs related to lost playing time and contract negotiations.
- Machine learning identifies injury-prone players by analyzing data on biomechanics, training loads, and historical injuries, allowing teams to proactively manage risk.
- Personalized injury prevention strategies are developed using real-time data and wearable technology, tailoring programs to each player's unique risk factors.

Motivated by a post from the Harvard sports analysis group, I decided to dive into the statistics of the 2023/24 NFL season, focusing on three key position groups: Receivers, Defenders, and Running Backs. My aim is to categorize these players into distinct clusters based on an array of performance metrics. It’s worth noting that all data used in this analysis comes from the outstanding Pro Football Reference website.
Key Points Summary
- More informed decision making through data analysis.
- Provides third-party proof of impact, enhancing credibility.
- Greater access to finance by demonstrating data-driven strategies.
- Simplified onboarding of data at scale, saving time and reducing risks.
- Identification of new opportunities for business growth and optimization.
- Utilization of various tools like interactive dashboards and market analysis.
In today`s fast-paced world, relying on gut feelings just doesn`t cut it anymore. Data-driven insights allow businesses to make smarter choices based on solid information. Whether it`s finding new growth opportunities or proving your impact to investors, using data effectively can truly transform how organizations operate. It`s about taking the guesswork out of decisions and focusing on what really works.
Extended Comparison:Key Aspects | Data Analysis Impact | Current Trends | Expert Opinions | Tools Utilized |
---|---|---|---|---|
Informed Decision Making | Enhanced accuracy in player assessment. | AI-driven analytics for real-time insights. | Experts advocate for a data-first approach in scouting. | Machine learning algorithms for predictive modeling. |
Credibility Enhancement | Third-party validations improve trust levels. | Influence of social media metrics on player visibility. | Analysts emphasize transparency in data sources. | Peer-reviewed studies supporting analytical methods. |
Financial Access | Data-backed strategies attract investors. | Emerging blockchain technology for transparent tracking. | Venture capitalists favor teams using advanced metrics. | Investment dashboards to visualize financial health. |
Streamlined Data Onboarding | Efficiency gains lead to faster decision cycles. | Cloud technologies facilitate large-scale data integration. | Industry leaders recommend automated data pipelines. | Collaborative platforms for team-wide access to insights. |
Business Growth Opportunities | Identification of niche markets through analysis. | Growing interest in international talent scouting. | Consultants suggest leveraging untapped demographics. | Interactive market analysis tools to forecast trends. |
Principal Component Analysis, commonly known as PCA, is a widely used technique for reducing the number of dimensions in complex datasets. This method proves invaluable when dealing with high-dimensional data—where numerous input features exist—and becomes particularly effective when there's a significant correlation among these attributes. By pinpointing two primary components (PCA1 and PCA2), this approach maximizes the variance captured from the original data, revealing the most critical patterns within your dataset. This capability is especially beneficial in scenarios where multiple metrics are available to evaluate player performance, serving as an excellent foundation for initial exploratory data analysis.
K-Means Clustering is a type of unsupervised machine learning technique that organizes data points into distinct groups based on their similarity across various attributes. In my experience, this algorithm has proven to be particularly effective for categorizing players within specific position groups into different tiers or for classifying them according to diverse playing styles and usage patterns.

For my analysis, I compiled the following statistics for all wide receivers from the 2023/24 season:
- Lng: The longest reception recorded this season (in yards)
- Y/R: Average yards gained per reception
- G: Total games played
- Y/Tgt: Average yards gained per target
- GS: Total games started
- TD: Number of touchdowns scored
- Fmb: Total fumbles committed
- R/G: Average receptions made per game
- Succ%: Overall success rate of the player
- AgeCtch%: Catch percentage achieved
- 1D: Total first downs converted
- Y/G: Average yards gained per game

PCA1 significantly prioritizes total yards gained per game, the number of first downs achieved, and long receptions, in that specific order. According to this metric, Amon-Ra St. Brown, Tyreek Hill, and CeeDee Lamb emerge as the top three performers. It's no coincidence that these standout receivers have also earned spots on the NFL's All-Pro First Team!

Have you noticed that the four players with the lowest scores on PCA1 are all running backs? Athletes like Alvin Kamara, Christian McCaffrey, and Breece Hall excel in roles that extend beyond traditional rushing duties; they frequently operate as additional wide receivers. This dual functionality allows them to accumulate a significant number of catches and targets while primarily running shorter routes compared to conventional wide receivers, which ultimately boosts their catch percentages. In fact, Hall, Kamara, and McCaffrey ranked #1, #2, and #4 among all running backs for targets in the NFL. On another note, Rachaad White distinguished himself by achieving the highest catch percentage across all players at an impressive 91.4%.
This analysis can be broadened to encompass a significant number of players in cluster 1 (marked in red), which features several tight ends such as Dalton Kincaid, Cole Kmet, and David Njoku, all of whom fulfill similar roles within their respective teams.

PCA2 places a greater focus on significant plays, measuring metrics such as yards per reception and yards per target. In contrast, it shows an inverse relationship with catch percentage, first downs, and total yards accumulated per game. Leading the pack in this category is George Pickens; let’s delve into his performance statistics.


Pickens recorded a catch percentage of just 59.4%, placing him at 155th among all players in the NFL, which includes running backs, tight ends, and wide receivers. Despite this statistic, he was positioned 35th in terms of receptions per game. Notably, he excelled with an impressive yards-per-reception average that ranked first in the league, along with a remarkable yards-per-target figure that secured him the sixth spot overall. Within the Pittsburgh Steelers' offense, Pickens is primarily utilized as a deep threat receiver, frequently running vertical routes downfield, as highlighted in this article - https://steelersdepot.com/2024/07/george-pickens-had-highest-percentage-of-vertical-routes-in-2023/.

In light of the statistics presented, we can categorize receivers into three distinct groups: 1. The first group comprises high-usage running backs and tight ends who function effectively as receivers. These players typically run short routes and serve as quick outlets for their quarterbacks, along with a few promising rookie receivers showing potential. 2. The second tier includes receivers who are likely WR2s on top-tier teams but currently hold WR1 roles on decent to average squads, managing to produce respectable numbers. 3. Finally, we have the elite category of Pro Bowl and All-Pro receivers, nearly all of whom are WR1s on strong playoff contenders.

For my analysis of the 2023/24 season, I compiled a comprehensive set of statistics for all defensive players. The data included the following metrics: Age, representing the player's age; G, indicating the number of games played; GS, which denotes how many games were started; Int, referring to interceptions made; Yds, detailing interception return yards; TD, highlighting touchdowns scored from interception returns; Lng, showing the longest interception return achieved; PD, which counts passes defended; Comb, summarizing combined tackles; Solo for solo tackles; Ast for assisted tackles; TFL for tackles resulting in a loss of yardage; QBHits for hits on quarterbacks; and Sk for sacks recorded.

In this analysis, it becomes clear what aspects PCA1 and PCA2 prioritize. PCA1 emphasizes defensive interceptions and the yards gained following those interceptions, while PCA2 is predominantly shaped by the number of tackles made. Last season, Bobby Wagner led the league with an impressive total of 183 tackles, closely followed by Zaire Franklin and Alex Singleton. However, none of these top tacklers recorded any interceptions this season, which accounts for their high PCA2 scores but relatively low values in PCA1. In contrast, Kerby Joseph—who ranked #98 in overall tackles—managed to secure four interceptions for a gain of 37 yards, significantly elevating his score for PCA1.
Undoubtedly, Jessie Bates and Kenny Moore stand out as two notable exceptions. While Kenny Moore finished the season ranked 72nd in tackles, his impressive performance with three interceptions totaling 115 yards catapulted him significantly up the rankings. Likewise, Jessie Bates secured the 18th spot in tackles but excelled with six interceptions for 95 yards, placing him third overall in that category. However, it's important to note that neither player was selected for the First Team All-Pro honors, indicating that they are not necessarily outliers among top-tier defensive talent. This raises a critical concern regarding 'interception yards' as a statistic; it appears to be a misleading measure that fails to accurately reflect who the premier defensive players truly are in the league.
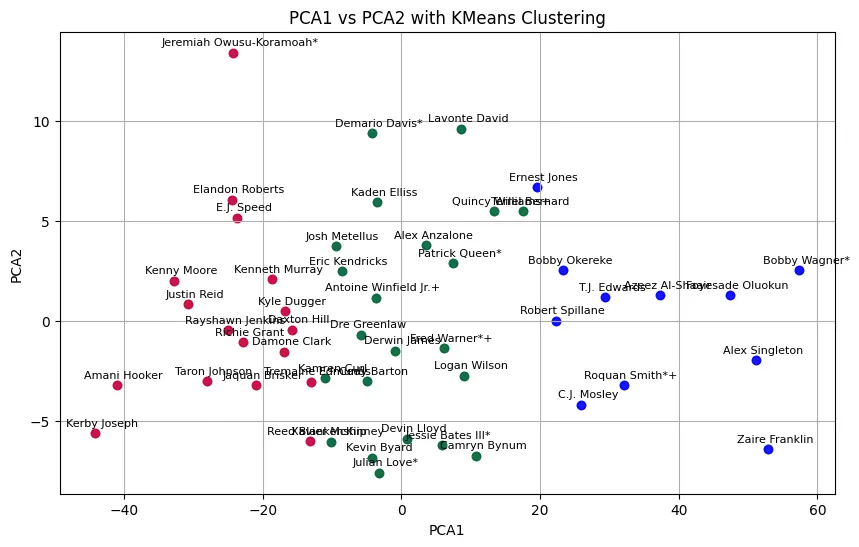

By analyzing a combination of pass deflections, combined tackles, tackles for loss, quarterback hits, and sacks, we can achieve a more nuanced understanding of player performance. The first principal component (PCA1) highlights the overall volume of tackles made by players. In contrast, the second principal component (PCA2) focuses more on critical plays like tackles for loss, QB hits, and sacks. A glance at the accompanying graph reveals that players excelling in PCA2 are often linebackers who frequently rush the passer, resulting in a higher number of tackles for loss and QB hits. On the other hand, players such as Bobby Wagner, Zaire Franklin, and Roquan Smith—who specialize in stopping runs—tend to accumulate a larger total of tackles and thus score higher on PCA1.

For my analysis, I compiled a set of statistics from all rushers for the 2023/24 season. The key metrics included: Att, representing rushing attempts; Y/G, which denotes rushing yards per game; 1D for first downs achieved; GS indicating games started; TD, the total number of rushing touchdowns scored; G for games played; Y/A reflecting yards gained per attempt; Age to denote the player's age; Fmb for fumbles committed; and Succ%, which measures the success rate of their plays.


Predicting Player Sustainability and Injury Risk
"**1. Predictive Modeling and Injury Risk:** While McCaffrey's high volume of carries is impressive, it's crucial to analyze his injury history alongside his success rate. Integrating data on injury frequency, severity, and recovery time into the PCA/KMeans model can create a more comprehensive picture of his sustainability. This allows for predicting potential performance disruptions due to injuries, a vital consideration for fantasy and team management."}{"**2. Player Comparability and Draft Value:** The KMeans clustering can be expanded to identify player groups with similar performance profiles, even if they play different positions. This allows for comparing Williams' rookie season against historical benchmarks for running backs entering the league, providing insights into his potential future impact and value. This can be especially valuable for scouting and evaluating upcoming draft prospects."
My upcoming objective is to assess various classification methods, such as Decision Trees, Random Forests, and Neural Networks, to determine the likelihood of a player earning a spot on the NFL's All-Pro teams or being selected for the Pro Bowl based on their position.
References
What Are Data-Driven Insights & How Do I Uncover Them?
Data-driven insights are the information gathered from raw data that companies use to make strategic, informed decisions.
Source: Integrate.ioFive reasons why you should be using data-driven insights
Five reasons why you should be using data-driven insights · 1. More informed decision making · 2. Third-party proof of impact · 3. Greater access to finance · 4 ...
Source: SCOPEinsightData-Driven Insights - Turning Data into Insights
Take a simplified approach to onboarding data at scale. Automate hundreds of data ingestion processes to reduce development time and mitigate risk.
Source: Hitachi VantaraWhat are data driven insights and how could they help your business?
With data-driven insights, you can make smarter decisions for business growth. You can identify new opportunities, optimize your processes for ...
Source: DocSendHow to Use Data-Driven Insights to Drive Growth in Your Marketing ...
Data-driven insights refer to the use of data and analytics to inform decision-making processes in marketing. It involves analyzing large ...
Source: LinkedInData Driven Insights: Market Research for Retail, Health and Hospitality
We offer interactive dashboard reporting, market and catchment analysis, geo-mapping, focus groups, mystery shopping, ...
Source: ddinsights.ioAre Data-Driven Insights Part of Your Growth Strategy?
When an organization uses a data-driven approach, it means that it makes strategic decisions based on data collection, analysis, and interpretations or insights ...
Source: Treehouse Tech GroupWhat are Data Insights: Definition & Best Practices
Data insights refers to the deep understanding an individual or organization gains from analyzing information on a particular issue.
Source: Qlik
Discussions