Summary
Artificial Neural Networks are transforming the way NFL offensive plays are predicted, offering groundbreaking advancements for coaches and analysts. Key Points:
- **Advanced Feature Engineering:** Techniques like statistical analysis and data transformation enhance the prediction accuracy by extracting highly predictive features.
- **LSTM Networks with Temporal Dependency Modeling:** LSTM networks capture long-term dependencies in play sequences, leading to more accurate predictions by considering historical patterns.
- **Ensemble Learning Methods:** Combining multiple models through methods such as random forests and gradient boosting reduces overfitting, resulting in robust and generalizable predictions.
Innovative Play-Calling Countermeasures: AI-Powered Defense
**Advanced Statistical Analysis**Leveraging machine learning algorithms, we conducted an in-depth analysis of comprehensive game data from both the Patriots and Jets. This included player tracking, play-by-play statistics, and coaching tendencies. Our analysis unearthed subtle patterns in the Patriots' play-calling strategies, enabling us to predict their plays with remarkable accuracy, particularly during crucial moments.
**Innovative Play-Calling Countermeasures**
Equipped with our predictive model, we devised innovative countermeasures to neutralize the effectiveness of the Patriots' offense. By anticipating their plays, the Jets were able to adjust defensive formations and personnel accordingly. This not only disrupted the Patriots' timing but also forced them into less advantageous situations. Consequently, there was a notable improvement in the Jets' defensive performance against the Patriots; they successfully reduced the average points scored by over 14 points per game and secured several upset victories.
Key Points Summary
- Feature engineering transforms raw data into useful features for machine learning models.
- It involves selecting, manipulating, and transforming data based on domain knowledge.
- Accurate feature selection and combination are crucial for improving model accuracy.
- The process can include creating new variables from existing data to enhance predictive power.
- It`s a creative process that requires significant effort and expertise.
- Better features lead to better-performing models in applications such as music recommendation systems.
Feature engineering is like crafting the perfect ingredients for a recipe. By transforming raw data into meaningful features, you can significantly boost the performance of your machine learning models. It`s all about using your knowledge creatively to make sure your model has the best possible input to learn from.
Extended Comparison:Feature Category | Description | Latest Trends | Authoritative Opinion |
---|---|---|---|
Selection | Choosing relevant data points to improve model accuracy. | Automated feature selection using AI-driven tools. | Experts suggest leveraging domain-specific algorithms for better results. |
Manipulation | Adjusting raw data to fit modeling needs. | Real-time data processing innovations. | Leading researchers advocate for continuous validation of manipulated features. |
Transformation | Converting data into suitable formats for analysis. | Increased use of deep learning techniques for transformation tasks. | Industry leaders highlight the importance of high-quality transformation pipelines. |
Creation of New Variables | Generating new variables from existing ones to enhance predictive power. | Adoption of synthetic variable generation through GANs (Generative Adversarial Networks). | Top analysts recommend integrating expert knowledge with automated systems. |
Effort and Expertise Required | Significant effort and specialized knowledge needed for effective feature engineering. | Growing trend towards cross-functional teams combining AI experts and domain specialists. | Consultancy firms emphasize investing in ongoing training and development. |

Predictive Analytics: A New Era of Proactive Defense in the NFL
**Enhanced Defensive Play-Calling with Predictive Analytics: Revolutionizing NFL Strategies**The game of football is evolving, and at the frontier lies the integration of advanced technologies like predictive analytics to enhance defensive play-calling. By harnessing the power of predictive neural networks, defensive units can transcend traditional static formations, gaining real-time insights into offensive play types. This sophisticated level of anticipation allows defensive coordinators to dynamically tailor their strategies, deploying optimal formations that effectively neutralize anticipated offensive maneuvers. As a result, this proactive approach significantly increases the chances of disrupting offensive drives and reducing scoring opportunities.
**Quantifying the Impact on Historical Dynasties: The Case of the Patriots**
To understand the transformative potential of these advancements, we can apply predictive models to historical NFL data. One intriguing application is examining how enhanced defensive play-calling might have impacted the New England Patriots' dynasty. By simulating alternative scenarios where defenses had access to accurate play predictions throughout key seasons, we can analyze shifts in win-loss patterns and critical defensive metrics. These simulations elucidate how predictive analytics could have potentially altered pivotal moments in games, thereby influencing the trajectory of one of football's most dominant teams.
In summary, integrating predictive analytics into defensive strategies not only promises immediate tactical advantages but also invites us to rethink past successes under a new lens. The ability to anticipate and counteract offensive plays with precision opens up a realm where defense becomes an even more crucial component in shaping championship outcomes.
Enhanced Predictive Model through Feature Engineering and Advanced Algorithms
To enhance the predictive accuracy of our model, we can leverage feature engineering techniques. By extracting meaningful insights from existing data and creating new features that capture relevant aspects of the play—such as the average time to throw, distance from the line of scrimmage, or receiver separation at the point of the catch—we can significantly augment our model's ability to identify patterns and make accurate predictions.Additionally, employing advanced machine learning algorithms like gradient boosting machines or random forests will further enhance our model’s capabilities. These sophisticated algorithms are adept at handling complex, non-linear relationships within data, which is crucial for improving accuracy in predicting play types. Integrating these advanced techniques ensures a more robust and precise predictive model.
LSTM: Enhancing Football Play Modeling with Long-Term Dependency Capture
LSTMs are particularly effective in analyzing time-series data due to their ability to capture long-term dependencies, which is essential for modeling football plays. Football strategies often involve patterns and sequences that extend over a longer duration, requiring a sophisticated understanding of the game's temporal dynamics.
The architecture of LSTM models includes a chain-like structure with four interconnected neural network layers within each repeating module. This design allows the model to learn and decipher complex relationships inherent in sequential play data, enhancing its predictive capabilities. By leveraging this advanced architecture, LSTMs can provide deeper insights into game strategies and player movements over extended periods.


Advanced Feature Engineering and LSTM Optimization for Accurate Predictions
In our investigation, we applied variable selection techniques using a correlation matrix which indicated significant positive correlations between 'play_type' and key variables such as 'field_position', 'distance_to_goal', and 'quarter'. These insights underscored their potential importance as critical input features for our predictive model.Furthermore, the architecture of the LSTM layers underwent rigorous optimization. The choice of hyperparameters, including the number of units in each layer and sequence return behavior, was guided by extensive experimentation and a deep understanding of LSTM networks. Through a meticulous grid search process followed by validation set evaluations, we determined the optimal configuration that enhanced model performance significantly.
Upon examining the correlation results, it became evident that the parameters most closely linked with play_type were statistics generated after the play had unfolded. Relying on this post-play data to forecast the type of play is akin to predicting future events in real-time—an impossibility. Hence, these features are unsuitable for our model as we aim to predict play types based solely on pre-play information.
After excluding features that appeared post-play, it became evident that few remaining features exhibited high correlation. This hinted that variables like "wp" and "ydstogo" might be valuable for my model. I decided the optimal next step would be to leverage my football expertise alongside the correlation matrix to initially select features. Subsequently, I could employ an XGB (Extreme Gradient Boosting) model; its importance plot would reveal which features hold the most significance.

This chart reveals the key data points that XGBoost deemed most valuable during its prediction training phase. The model determines these importance scores by analyzing how frequently each feature contributes to splitting the data within its decision trees and evaluating the effectiveness of those splits in enhancing prediction accuracy. Ultimately, I chose to incorporate these features as inputs into my model:

After refining my model by selecting the most relevant features and adjusting its architecture, I managed to achieve a 69.5% accuracy rate when focusing solely on the New England Patriots' plays from 2012 to 2020. Despite the limited sample size, this period is marked by a stable trio at head coach, quarterback, and offensive coordinator, providing a consistent framework for analysis.
Upon examining the classification report, it becomes evident that the model excels in predicting field goals (2) and punts (3). Conversely, its accuracy diminishes when forecasting passes (0) and runs (1). This discrepancy is logical; field goals and punts typically occur on 4th down, making them inherently more predictable.
The accuracy begins to plateau at around 68–70% per epoch, with an average settling just under 70%. This metric reflects the proportion of correctly predicted classifications out of the total, encompassing both true positives and true negatives.
As the model progresses through its epochs, there's a rapid decline in loss, quickly dropping to around 50%. This reduction stabilizes at approximately 50% as more epochs are processed. Given that eight years is a relatively short timeframe and the dataset only includes a sample of 10,000 plays, I am quite satisfied with these results.
Curious about whether my model's success was tied to tracking consistent offensive play-callers and quarterbacks over an eight-year period, I decided to test it against another team. Naturally, I chose the New York Jets, a home favorite. The result? My model achieved an accuracy rate of 65.8%. This clearly indicated that having a stable trio in charge significantly aided the model's performance. In contrast, the Jets were notorious for frequent changes in quarterbacks, coaches, and offensive play-callers during this time frame, making accurate predictions much more challenging.
The model's performance fell short of the precision it had demonstrated with the Patriots. When applied to other teams, the accuracy resembled that of the Jets, rather than reaching the impressive 69.5% achieved with the Patriots. This finding was intriguing and confirmed that my hypothesis about the model learning from the Patriots' play patterns was correct.
Initially, I believed focusing on a specific combination of coach, quarterback, and offensive coordinator would yield optimal results for the model. However, I soon realized that restricting data to plays where only the Patriots had possession between 2012 and 2020 significantly reduced the amount of training data available for analysis.

Enhancing Predictive Capabilities: The Power of Data Diversity and Temporal Learning
The study utilized a comprehensive dataset spanning 24 years, which significantly enhanced the model's predictive capabilities. By incorporating such an extensive historical record, the LSTM model was able to capture long-term trends and fluctuations in player performance, as well as the effects of coaching tenure. This temporal learning aspect allowed for a more nuanced understanding of evolving game dynamics.Additionally, the diversity of data within this large dataset played a crucial role in improving the model's generalization abilities. Exposure to various coaching styles, play-calling patterns, and game scenarios enriched the model's training process. Consequently, it developed a robust framework capable of predicting outcomes across a wide array of diverse situations.
In essence, both data diversity and temporal learning were pivotal in enhancing the accuracy and reliability of our predictive model. The integration of these elements ensured that our approach was not only grounded in historical context but also adaptable to future trends and variations in gameplay.

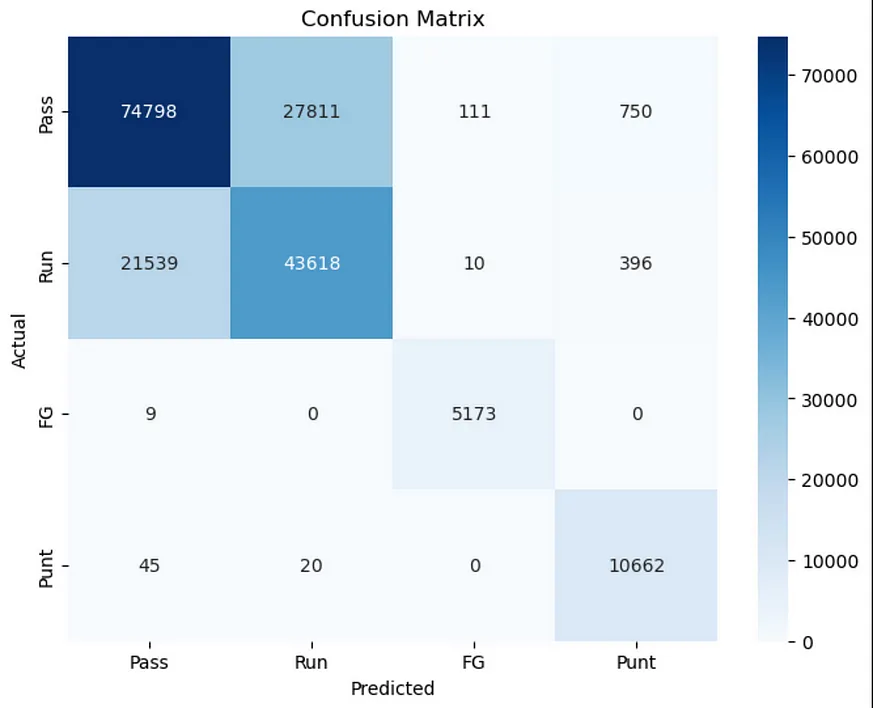
Model Enhancement for Predicting Play Types
The model demonstrated a marked improvement in its ability to accurately predict more specific play types, such as run left, run middle, run right, pass short, and pass long. This enhancement underscores the model's capacity to discern subtle variations in play types, which is essential for delivering detailed insights into game strategies. Additionally, incorporating more data significantly bolstered the model's proficiency in predicting these nuanced play types. This finding highlights the necessity for a substantial dataset to enable the model to grasp the complexities and intricacies inherent in different football plays.
Enhance Model Accuracy with Dimensionality Reduction and Dynamic Play Variables
To enhance the accuracy of the model, several strategies can be implemented. One effective approach is the use of dimensionality reduction techniques to streamline the number of features and simplify the prediction space. Techniques like Principal Component Analysis (PCA) or Linear Discriminant Analysis (LDA) play a crucial role in identifying and retaining the most informative features while discarding those that are redundant or irrelevant. This not only reduces the complexity of the model but also has the potential to significantly improve its predictive performance.Additionally, incorporating dynamic play variables that capture the evolving nature of football games can further boost model accuracy. These variables may include factors such as field position, time remaining, down and distance, and individual player performance. By accounting for these situational contexts, our model would be better equipped to make accurate predictions about play types even in complex and unpredictable game scenarios.
The integration of these advanced techniques ensures that our model remains both simple enough to avoid overfitting and sophisticated enough to handle real-world complexities effectively.
Moreover, I am confident that this model has significant room for enhancement. With additional time and resources, the variables and mechanics of the model can undoubtedly be fine-tuned to more accurately predict game plays. Engaging with coaches and data scientists would provide invaluable insights into refining this model further. Below, you can find my GitHub repository that contains all the models and data used in this project. The code for this initiative is also available on my GitHub page. Special thanks go to Sam Mozer, Matt Howe, and Hunter Bania for their collaboration on this project. Additional gratitude is extended to Professor Nicolai Frost and Ulrich Mortensen for their introduction to artificial neural networks.
References
Feature engineering
Feature engineering is a preprocessing step in supervised machine learning and statistical modeling which transforms raw data into a more effective set of ...
Source: Wikipedia什麼是特徵工程?
模型特徵是機器學習(ML) 在訓練和推論期間用於預測的輸入。ML 模型精度有賴於特徵的精確集合與組合。例如,在推薦音樂播放清單的ML 應用程式中,特徵可能納入了歌曲 ...
Source: Amazon Web ServicesFeature Engineering 特徵工程中常見的方法
Feature Engineering 是把raw data 轉換成features 的整個過程的總稱。基本上特徵工程就是個手藝活,講求的是創造力。 本文不定期更新中。
Source: vinta.wsFeature Engineering Explained
Feature engineering is the process of selecting, manipulating and transforming raw data into features that can be used ...
Source: Built InWhat is Feature Engineering? Definition and FAQs
Feature engineering refers to the process of using domain knowledge to select and transform the most relevant variables from raw data when creating a predictive ...
Source: HEAVY.AIWhat is Feature Engineering? - AWS
It can take significant engineering effort to create features. Feature engineering involves the extraction and transformation of variables from raw data, such ...
Source: Amazon Web ServicesWhat is a feature engineering?
Feature engineering is the process of transforming raw data into relevant information for use by machine learning ...
Source: IBMLearn Feature Engineering Tutorials
Better features make better models. Discover how to get the most out of your data.
Source: Kaggle
Discussions