Summary
Can a single statistic accurately predict college baseball runs? This article delves into the power of advanced data analytics in improving run predictions for college baseball. Key Points:
- **Advanced Statistical Models Enhance Run Prediction**: JOPS (Adjusted On-Base Percentage Plus Slugging) is a reliable metric that captures both batter skill and game environment, enhancing offensive production predictions.
- **Overcoming Data Biases for Accurate Predictions**: Techniques like bias-variance trade-offs and cross-validation ensure models balance complexity and generalization, preventing over-reliance on specific datasets.
- **Predictive Model Precision in Different Leagues**: The precision of run prediction models varies between MLB and Division 1 teams due to factors like sample size, player skill disparity, and game intensity.
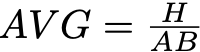
Key Points Summary
- Complete source for baseball history, including major league stats, awards, records, and more.
- Official player hitting stats, home run leaders, batting average, OPS, and other stat leaders.
- Statistical analysis for both Major League Baseball (MLB) and Minor League Baseball (MiLB).
- Fantasy baseball relies heavily on standard stats like batting averages and home runs.
- Current MLB player stat leaders include notable performances in categories like HRs and RBIs.
- ESPN is a key resource for up-to-date MLB Regular Season stat leaders.
If you`re a baseball fan looking to dive deep into the sport`s rich statistical world or just need the latest player performance data for your fantasy league, there are comprehensive resources available. From historical records to current season leaders in various categories such as home runs and batting averages, you can find everything you need. Websites like ESPN offer detailed statistics that keep fans informed throughout the season.
Extended Comparison:Source | Coverage Area | Key Metrics | Notable Features |
---|---|---|---|
Baseball-Reference | Historical and Current MLB, MiLB | Major league stats, awards, records, player hitting stats | Comprehensive historical data, detailed player profiles |
ESPN | Current MLB Regular Season | Home runs (HRs), Runs batted in (RBIs), batting average (BA) | Real-time updates, expert analysis |
Fangraphs | MLB and MiLB Statistical Analysis | Advanced metrics like WAR (Wins Above Replacement), wRC+ (Weighted Runs Created Plus) | In-depth sabermetrics, customizable leaderboards |
MLB.com | Official MLB Stats | Standard stats: HRs, RBIs, BA; Advanced stats: OPS (On-base Plus Slugging) | Official source with video highlights and player news |
FantasyPros | Fantasy Baseball Stats for MLB | Batting averages, home runs (HRs), RBIs | Specialized for fantasy baseball strategies and projections |


In 1980, Bill James introduced the term "sabermetrics," setting off a rapid evolution in baseball statistics. In this article, we'll delve into two of the more popular and user-friendly advanced metrics within Division 1 college baseball.
First up is on-base plus slugging (OPS), which is calculated by adding on-base percentage (OBP) and slugging percentage (SLG). It's essential to note that SLG, which ranges from 0 to 4, carries more weight than OBP, which ranges from 0 to 1. This raises the question: does this weighting make sense? Given that scoring runs is the ultimate goal in baseball, let's examine how effectively a team's OPS correlates with their average runs scored per game.
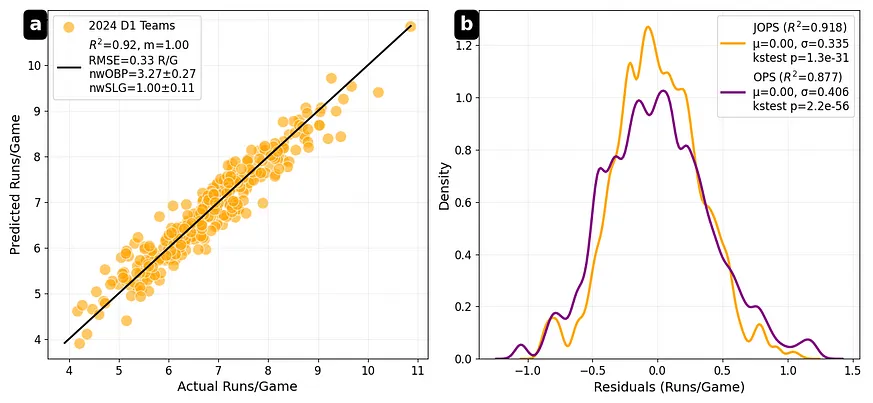
Impact of Statistical Tools and Innovations on Baseball′s Run Environment
One intriguing aspect of baseball statistics is the use of JOPS (Joe Haumacher's On-base Plus Slugging) as an indicator of the run environment. Unlike OPS, JOPS places a heavier emphasis on OBP (On-base Percentage), which can reveal underlying factors in different leagues or seasons. For instance, the heightened importance of OBP in JOPS compared to OPS in D1 implies that fielding in D1 is generally less reliable than in MLB. This unreliability increases the likelihood of runners scoring without needing extra base hits.Furthermore, changes within a league can significantly affect its run environment. A notable example is what happened in D1 after the 2021 season with the widespread implementation of barrel testing. Barrel testing measures bat performance, particularly focusing on how well a bat can hit balls at high exit velocities and optimal launch angles. The introduction and extensive use of this technology likely resulted in more effective bats, thus contributing to an increase in runs scored within D1 games.
By examining these elements—JOPS's unique weighting and technological advancements like barrel testing—we gain deeper insights into how statistical tools and innovations shape the dynamics of baseball across different contexts.

A slight increase in complexity could significantly enhance both OPS and JOPS metrics. OPS and JOPS currently incorporate SLG, which intuitively—but somewhat arbitrarily—assigns weight to each type of hit based on total bases earned. But what if we assigned a unique weight to each on-base outcome? This is where the Weighted On-Base Average (wOBA) comes into play, as detailed in Tango, Lichtman, and Dolphin's 2006 publication "The Book." This statistic aims to provide distinct weights for every possible on-base event.

JOPS: A Robust and Effective Metric for Offensive Production
In both Division 1 college baseball and Major League Baseball (MLB), JOPS has demonstrated consistent reliability as a metric for predicting Runs/Game. It has outperformed wOBA in certain years and matched its performance in others. This consistency underscores the robustness of JOPS as an analytical tool.Furthermore, the notable similarity between JOPS and OPS within MLB highlights the effectiveness of JOPS as a comprehensive indicator of offensive production. This resemblance suggests that JOPS can serve as a comparable alternative to the widely used OPS metric, providing valuable insights into player performance and team strategy.
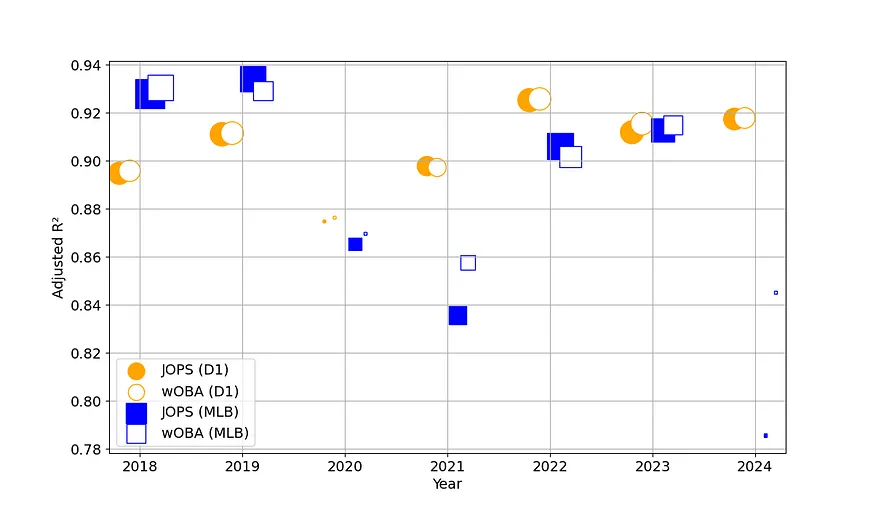

Addressing Overfitting Through Bias-Variance Trade-off and Cross-Validation
**Addressing Overfitting: Bias-Variance Trade-off**One of the critical challenges in machine learning is overfitting, where a model becomes too intricately tuned to the training dataset and fails to perform well on new, unseen data. To mitigate this issue, it is essential to find an optimal balance between model complexity and accuracy. This involves careful tuning of the number of parameters within the model and applying regularization techniques that penalize overly complex models. These strategies help enhance the generalization capabilities of the model and ensure that it performs well on both training data and new data.
**Cross-Validation for Robust Model Evaluation**
A widely adopted method to combat overfitting is cross-validation, which serves as a robust tool for evaluating a model’s performance. Cross-validation works by splitting the training data into several subsets and then iteratively training the model using different combinations of these subsets. During each iteration, one subset is used as validation data while the others are used for training. This process provides a more reliable estimate of how well the model will generalize to unseen data by assessing its performance across various iterations. Moreover, cross-validation helps in identifying the most suitable complexity level for a given dataset, contributing significantly to building a well-generalized and efficient predictive model.

I am set to conduct another experiment aimed at assessing the relative effectiveness of JOPS and wOBA in forecasting runs scored. The standard approach for this analysis is cross-validation, which involves training the model on a subset of the data and then evaluating its accuracy with the remaining portion. While I acknowledge Samuele Mazzanti's insightful critique on the limitations of cross-validation, I intend to proceed cautiously.
Figure 5 presents a wealth of information, so I'll aim to avoid getting bogged down in the minutiae. Beyond the standard random trials depicted in gray, I have also organized annual trials showcased in red and purple to assess how poor the model's performance can be. The core concept here is to identify the optimal model, whether JOPS or wOBA, for a specific year and then utilize that model to forecast Runs/Game for subsequent years (as illustrated in Figure 5; Xval by Year). As highlighted in Figure 2, this test may yield suboptimal results, particularly for D1, due to year-to-year fluctuations in model weights. Nonetheless, this challenge mirrors our real-world scenario: we must predict future outcomes based on data from previous years!

The Precision of Predictive Models for Runs/Game: MLB vs. D1 Teams
When evaluating the precision of predictive models for Runs/Game, it is evident that the model performs with greater accuracy for MLB teams compared to D1 teams. This conclusion is drawn from analyzing the standard deviation of the residuals, where MLB exhibits a value of 0.51 while D1 shows a higher value of 1.26.Furthermore, in assessing different predictors such as JOPS and wOBA, we find that JOPS consistently outperforms wOBA in both leagues despite its relative simplicity. The coefficient of determination for JOPS stands at 0.33 in D1 and 0.15 in MLB, whereas wOBA scores slightly higher at 0.35 in D1 but lower at 0.16 in MLB. This highlights that even though wOBA is a more complex model, JOPS proves to be a better predictor of Runs/Game across both divisions.
These insights underscore the importance of not only choosing precise models but also considering simpler metrics like JOPS which can sometimes yield superior predictive power over more intricate alternatives like wOBA.
On one side, JOPS stands out as an exceptionally straightforward yet effective metric in both Division 1 college baseball and Major League Baseball. It explains over 90% of the variance in runs scored per game with merely a single adjustable parameter (Figures 1–3). However, whether you opt for JOPS or wOBA, predicting a team's Runs/Game within 25-30% accuracy of the actual runs per game only succeeds about 68% of the time. If you were to ask me which statistic I would choose to build a D1 lineup, my answer would undoubtedly be JOPS. Nevertheless, I'm eager to put other advanced statistics through their paces in D1 college baseball. Next on the agenda: integrating park factor and batted ball metrics into our analysis.
References
Baseball-Reference.com: MLB Stats, Scores, History, & Records
Complete source for baseball history including complete major league player, team, and league stats, awards, records, leaders, rookies and scores.
Source: Baseball-Reference.com2024 MLB Player Hitting Stat Leaders
The official source for player hitting stats, MLB home run leaders, batting average, OPS and stat leaders.
Source: MLB.comFanGraphs Baseball | Baseball Statistics and Analysis
Baseball statistics for Major League baseball and Minor League baseball with statistical analysis, graphs, and projections.
Source: FanGraphsBaseball statistics
Baseball statistics refers to a variety of metrics used to evaluate player and team performance in the game of baseball. 1911 baseball card showing Jake ...
Source: WikipediaMLB Player Stat Leaders, 2024 Regular Season
The 2024 MLB Regular Season All MLB Player stat leaders on ESPN. Includes stat leaders in every category from home runs and batting average to strikeouts ...
Source: ESPNStandard Stats | Glossary
Standard stats are widely used by those who play fantasy baseball. A typical fantasy baseball league will use the following statistics: For Hitters: Batting ...
Source: MLB.comMLB Stats & Leaders
Batting ; 1. Kansas City. B. Witt Jr. 12 HR, 53 RBIs .314. AVG ; 2. Minnesota. C. Correa. 8 HR, 36 RBIs .308. AVG ; 3. NY Yankees. J. Soto. 18 HR, 56 RBIs .305.
Source: Yahoo SportsMLB Batting All MLB All Positions Stat Leaders, 2024 Regular Season
ESPN is the place for MLB stats! Discover the All MLB Batting All Positions stat leaders of the 2024 MLB Regular Season.
Source: ESPN
Discussions